

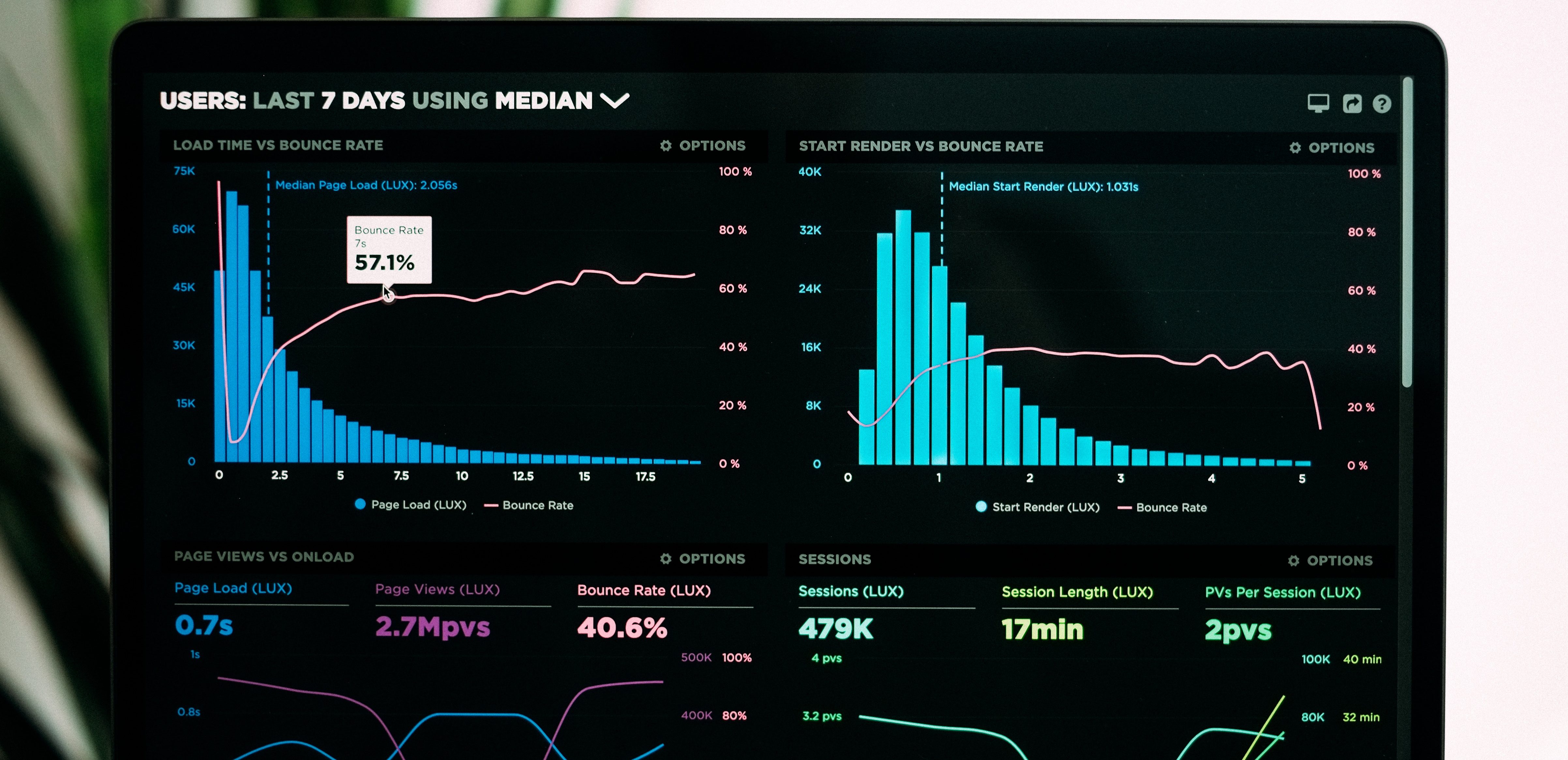
Advice On How To Make Your First Analytics Hire
Recently, I’ve been working with a few of our portfolio companies to help them think about how to recruit and interview their first analytics hire. As the former Head of Product at Blue Apron where I also built and led our Analytics & Data Science team (I know enough SQL to be dangerous), I have an intuitive sense for the “when” and “what” of this role.
Given the common challenges encountered by founders as they navigate through this hiring decision, I thought I’d outline some of my thoughts below that can hopefully serve as a simple framework when it comes to making the first Analytics hire at your startup.
Analytics vs. Finance — what’s the difference (and what do you need)?
Many founders confuse the two as they both involve lots of numbers. In reality, these two domains require quite different skill sets (more on this later). Below is a simple way I find helpful in figuring out what organizational capability you’re most urgently missing.
- Analytics is about designing, reporting, and leveraging operating metrics to aid strategic and functional decision-making. Examples of this work could range from defining how CAC and LTV (historical and predictive) are calculated for the business, how to think about marketing ROI and payback period by cohort, to designing the right parameters and analytics framework for A/B testing and designing metrics such as OTIF (“on time in full”) for fulfillment operations. These are operating metrics because they are not part of GAAP financial statements, even though they are critical for everyone from the CEO to marketers and PMs to make data-informed decision on a daily basis.
- Finance is about reporting on historical performance and future planning through the lens of financial metrics. This is a bit of an oversimplification as there are many sub-disciplines within finance — accounting/controller, FP&A, demand forecasting, etc. — but at a high level the work output here is usually historical or forecasted view of the business conveyed through GAAP financial metrics/statements, done in monthly, quarterly, or annual cadence.
Let’s say that you’re trying to figure out whether your marketing spend is efficient across channels, looking at your monthly Income Statement (financial metrics) which has revenue and marketing expenses rolled under SG&A for a given month doesn’t really tell you much; you’re much better off slicing the data by monthly customer cohort and observe the relationship between CAC vs. cumulative margin LTV for a given channel (operating metrics).
Conversely, if you’re trying to understand when you’ll run out of cash, you need to put together a forecasted Cash Flow Statement to understand how your working capital needs and fixed/variable cash expenses impact your monthly burn.
Of course, Analytics and Finance are never cleanly separated and these two teams usually collaborate closely (especially when it comes to forecasting/budgeting). In general, when a startup is young with relatively simple operations, Finance is an easier function to outsource as the work requires less company-specific knowledge, agility, and database access (you’re closing the books monthly, not monitoring marketing campaigns or product experiments weekly).
When to make your first analytics hire
In the very early days, founders are probably wearing the Analytics hat, or worse, nobody is looking at the data and making decisions blindly. Being a data analyst in addition to being the founder is a lot of work — you’re writing SQL queries, manipulating the data sets in excel and creating graphs to make sense of them, defining what metrics to look at in your weekly dashboard, and implementing some sort of automated report generation. You might be able to get your engineering team to do some of the above, but you most likely can’t offload the strategic thinking around “what metrics/ways to look at the data is the best to answer X question” until you have someone focused on analytics full-time.
So when should you start thinking about your first analytics hire? The moment you feel that the sheer number of decisions that should be data-informed are either getting rushed without such data or severely bottlenecked, hurting the speed of execution and putting the business at risk.
What to look for in your first analytics hire
Similar to Product Managers, a strong Analytics hire requires a diverse set of skills. It’s close to impossible to find such “unicorn” who excels at all of the below — you should prioritize and focus on the ones that your existing team lacks the most (or the ones that you need to offload/delegate the most).
- Analytical intuition: In my opinion, analytical intuition is probably the most important, and hardest to find, trait. It means the ability to ask 2nd- and 3rd-order questions through data manipulation and derive interesting insights out of seemingly unwieldy, large sets of numbers. Someone with strong analytical intuition can become a strategic thought partner with the leadership team and functional leaders.
- Technical proficiency: This usually means one’s ability to navigate the database and write SQL queries/Python/R scripts to retrieve the data in question and doing advanced data manipulation. The difference in technical proficiency here could mean whether your analyst can “self-serve” without bothering the engineering team (who would rather focus on building the product) while still being effective. I’ve seen candidates who came from a non-technical background and started out with only excel skills but eventually picked up the technical skills on the job (at least basic SQL) — you just have to be aware of the tradeoff.
- “Data science” knowledge: This could range from advanced knowledge around statistical methodology and predictive modeling to more technical understanding of machine learning principles. This skillset is usually not required / less important until later stage of the business when you’re wrestling with more complex questions, such as “what are the predictive indicators of churn?”, “what’s the forecasted LTV of given customer cohort?”, or the need to build dynamic demand forecasting models.
- Financial acumen: It is true that an Analytics hire looks pretty different than someone in the Finance function, but it is also true that the most effective analysts have strong finance fundamentals, for two reasons. For one, they end up serving as liaison between the raw data that sits in your production database (which represents your operating reality) and the Finance team. Additionally, understanding how financial reporting is usually presented (e.g. YoY, MoM growth rates, % revenue by customer segment) to concepts such as margin and working capital is tremendously useful when determining what operating metrics to focus on as a business.
Candidate archetypes — where to look for them
The tricky thing here is to translate the above skillset matrix into pattern-matching “academic and work experiences,” and your first inclination might be targeting who are currently on the Analytics or Data Science teams at other companies.
Based on my experience, I would strongly encourage you to stay open-minded when it comes to candidate archetypes. Over the course of building out the 15-person Analytics team at Blue Apron, I saw some of our best analysts coming from “non-traditional” backgrounds — from ex-consultants and bankers or those who used to work in quantitative research or at hedge funds, with degrees from physics to finance to engineering to PhD in statistics.
Look to hire someone who can bring knowledge in the areas your company needs help with most, coupled with a proven track record of learning quickly and picking up new skills on the job. When you don’t have a large team and are just making your first Analytics hire, keep in mind which areas you can afford to be patient in and where you need immediate contribution.
Good luck!