

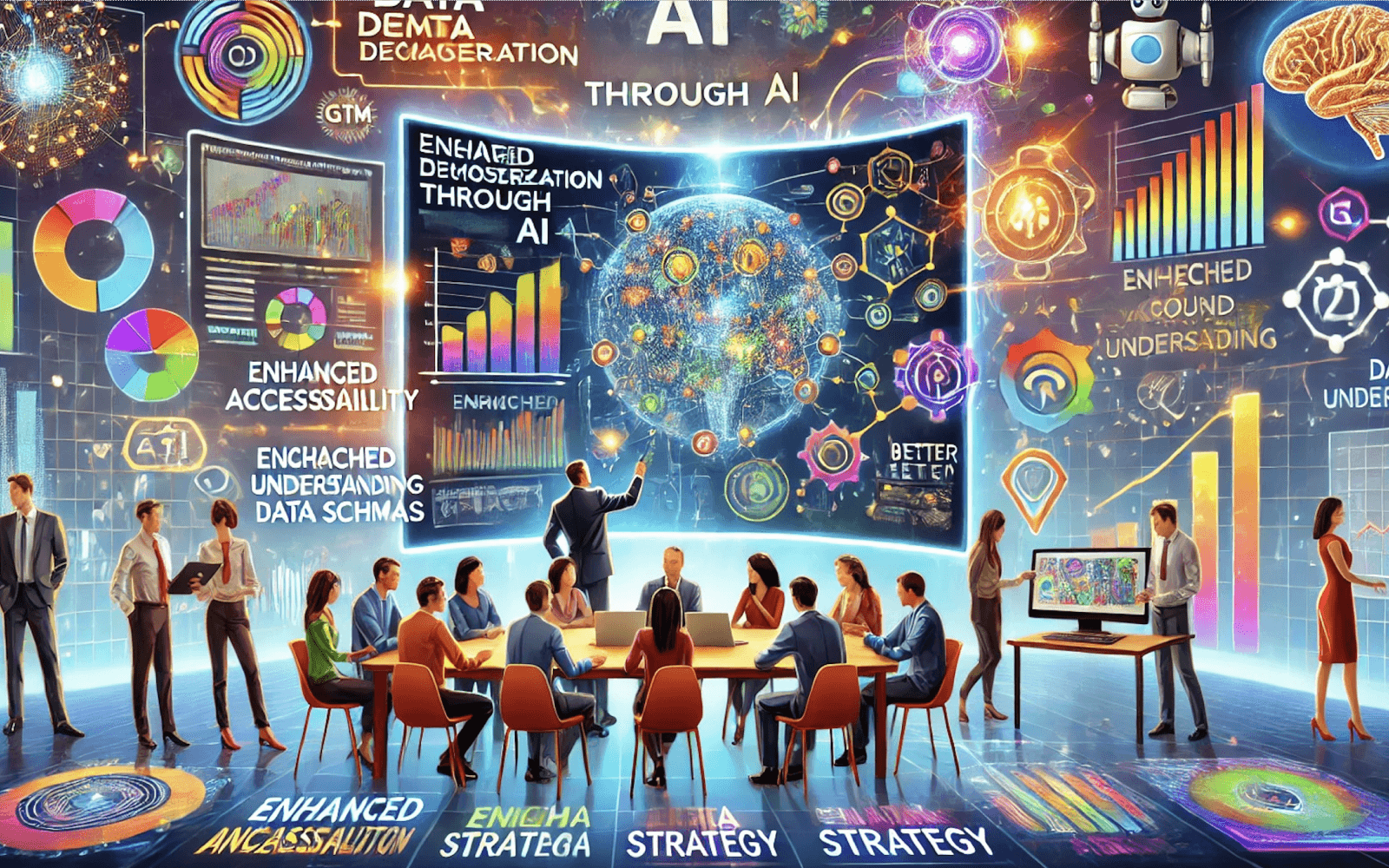
Advancing Data Democratization in the Age of Generative AI – Opportunities and Challenges
The concept of data democratization, i.e., increasing ease of access and speed to high-quality data insights across the organization, has been a thematic area of interest given its pervasive impact on the everyday lives of knowledge workers. The advancement of LLMs has opened up new technical possibilities, and we’ve met many founders over the past 18 months rushing to build and innovate in this space. Here, I want to share a few observations and thoughts on the opportunities presented by this technology, as well as some of the persistent challenges founders will need to continue to wrestle with.
Opportunities
1. Enhanced Data Accessibility through Natural Language Interfaces
Data insights are not technical in nature, but historically, the interface to interact and produce data insights is. Generative AI presents the opportunity to leapfrog the technical interface by providing the ability to interface with natural language. Users no longer need to understand SQL to converse and manipulate data. This removes data science/data engineering teams as the bottleneck and truly democratizes access to data insights in terms of reach and speed.
2. Enriched Schema Understanding
Historically, the advancement around automating data insights has been bottlenecked by the disconnect between business context and the (often poorly designed) data schema, which usually lacks proper documentation. Generative AI can leverage qualitative, unstructured data sources to enhance schema understanding and potentially automate schema documentation. This sets the foundation for accurate querying and presentation of the data question at hand.
3. Conversational Interfaces for Better Data Queries
AI-driven conversational interfaces allow for “few-shot prompts” that guide users in formulating the right data questions. This is particularly valuable for users unfamiliar with the underlying data structure, akin to having a dialogue with a data scientist to clarify and refine their queries. That being said, framing a complex data question the right way is hard, especially for non-technical users who are not familiar with the data schema/architecture. I believe this is an area where innovations around both how to interact with the models and how to present the user experience have the potential to solve, with UX iterations on the come.
Challenges
1. GTM – Challenges Abound Whether You’re Supplementing or Replacing Data Teams
If the product is capable of supplementing but not completely replacing data scientists/data analysts, then the buyer/key stakeholder is probably the data team, who could be critical in a non-perfect product that does not handle advanced data problems or edge cases well.
Alternatively, you aim to be the data team alternative. To start, you can go after young companies earlier in their data team journey and try to follow the low-end disruption path. However, targeting companies early in their data journey to replace data scientists altogether can be difficult due to the narrow window between recognizing the need for data expertise and hiring a data leader.
The other consideration is the data engineering and infrastructure work required to get the data ready for querying and presentation. If the company still needs to hire a data engineering team to do all the work to set up the data stack, then they might not find outsourcing just the data insights piece compelling.
2. GTM – The Pain Doesn’t Live with the Budget
Sometimes the pain aligns with the budget in that data teams feel the pain of overwhelming data requests from other teams, and there’s pressure from leadership to deliver. This is usually a more straightforward sale if you can justify the ROI around efficiency gain of the existing data team headcount (notwithstanding the challenge above).
However, true data democratization really unlocks the speed and quality of decision-making for everyone else in the organization, from the exec team to key functional teams such as sales, marketing, and product. The VP of Marketing or Sales can be your champions, but if a company is large (and thus the pain around the lack of speed in delivering data insights is more pronounced), then it’s more likely a data team is already established, and this team ends up being both the final decision maker and budget source.
3. Complexity Around Understanding Data Schemas
Even though generative AI presents some opportunity in this area, being able to accurately cover 100% of the data is hard, especially for first-party data not coming from the standard SaaS tools (thus more standard schemas). AI might get you to 80% accuracy, but the human lift required internally to correctly map/document the remaining 20% could prove to be too much of a lift and becomes a barrier for either adoption or accurate output.
4. Human Habits are Hard to Break
The promise of data democratization is all about speed and agency. Assuming we can overcome all the technical and GTM challenges, a successful data product allows everyone from the CEO to SDR to ask complex data questions and get fast answers, versus having their data requests sitting in the queue for weeks and static dashboards that they can’t drill down or manipulate. However, “throwing your question to the other side of the fence” is potentially the much easier (and more comfortable) thing to do, versus learning how to talk to an “AI data analyst.”
We tend to overestimate technical advancement but underestimate the corresponding behavioral changes required to take advantage of such advancement, and I think there’s potentially a similar trap in this problem space.
As with many other verticals and domains, I think we are still extremely early in exploring how to best harness generative AI to unleash access to data insights. If you’re thinking and building in this problem space, I’d love to chat.